AI has changed the game, and the generative enterprise will take center stage
Generative AI’s disruptive impact on every industry brings with it new opportunities for organizations to seize and new risks they must mitigate as they forge their strategy for the future.
In this year’s Tech Trends report, we examine three trends to help seize the opportunities, and three trends that will help mitigate risks.
Generative AI will drive the agenda
As AI reshapes industries and business operations, IT and business leaders must adopt a strategic and purposeful approach across six areas to ensure success.
Seize Opportunities:
- AI-driven business models
- Autonomized back office
- Spatial computing
Mitigate Risks:
- Responsible AI
- Security by design
- Digital sovereignty
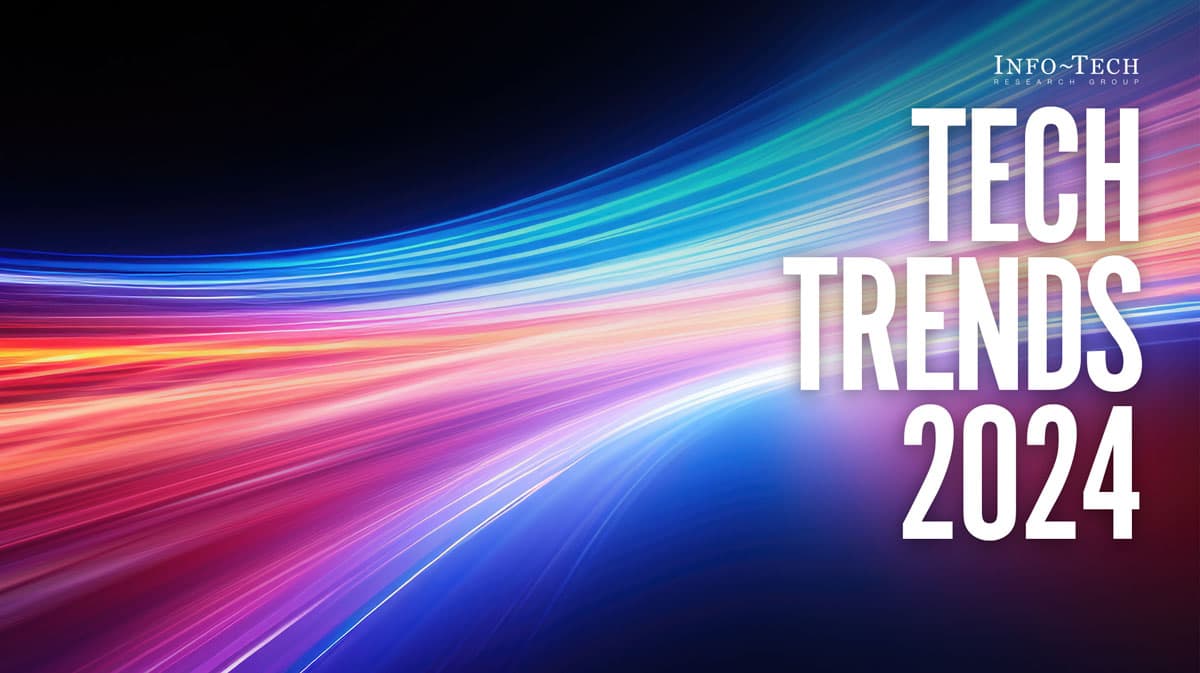
Table of Contents
Seize Opportunities
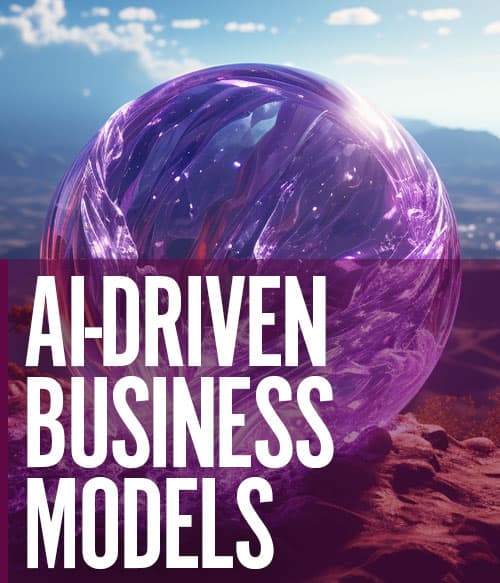
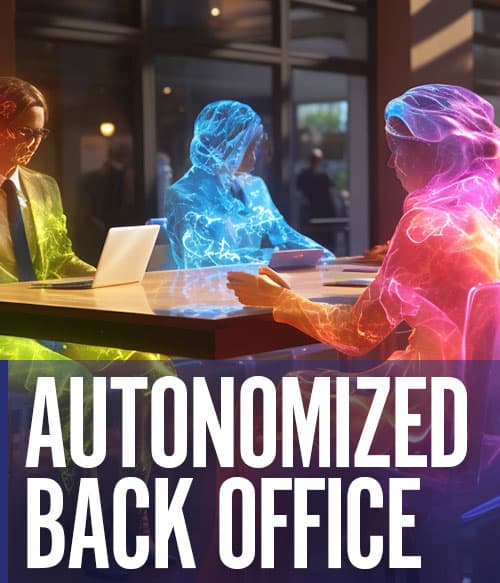
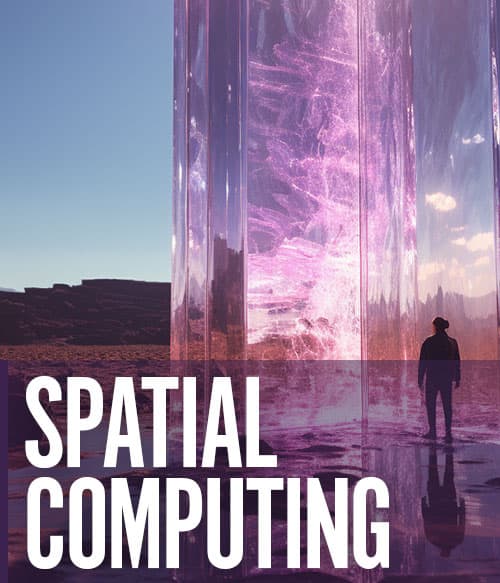
Mitigate Risks
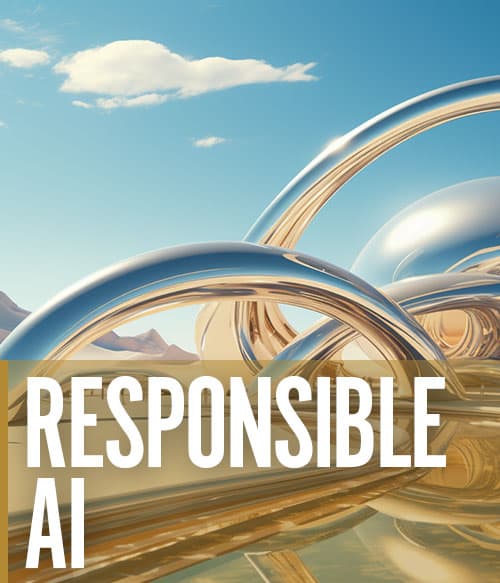
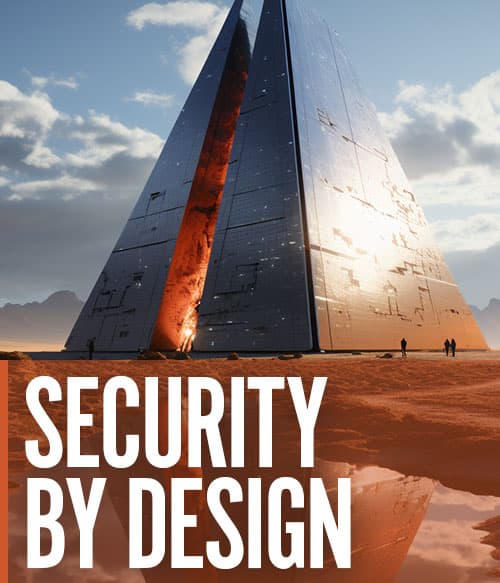
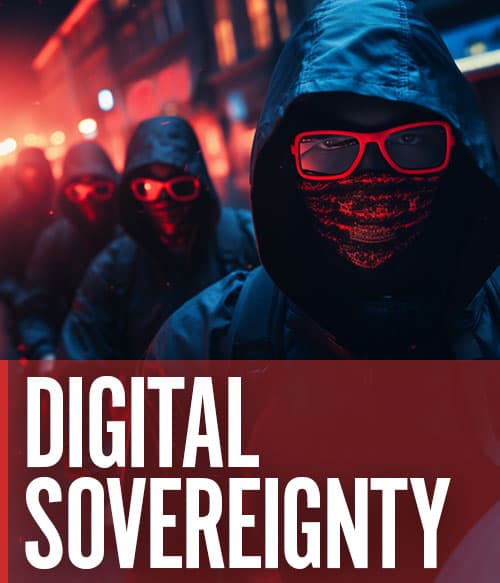
Introduction
What Moore’s
law can teach us about our relationship with AI
When Gordon Moore made a prediction in a 1965 paper, he couldn’t have fathomed that it would become the most famous law of computing. Moore’s law originally stated that computer processing power would double every two years and that this trend would last for at least 10 years (Moore, 1965). Afterward, he was proven correct as integrated circuits became more efficient and less expensive at an exponential rate. The trend lasted beyond Moore’s predicted 10-year span, holding true for decades.
In March 2023, Moore died. Whether Moore’s law outlives him or not is a matter of debate. Some say we are nearing the physical limits of the number of transistors that can be packed into a silicon wafer. But whether or not the concept still applies to chips is not as important as the broader lesson learned about the feedback loop created between humanity and technology. It’s one of exponential advancement, and it’s why Moore’s law is now commonly used to describe many different advances in computing beyond processing power.
Once made, Moore’s prediction turned into a goal – one that chip designers strove for as their North Star of progress. Designers used high-performance computing (HPC) to augment their designs, solving mathematical and engineering problems required to more densely pack transistors together. Hence, the demand for HPC increased. This supported the design of devices that preserved Moore’s law, leading to even more powerful HPCs, and so on (HPCWire, 2016). This feedback loop eventually produced today’s nanometer-scale chips that power our smartphones and wearables.
A relationship that produces benefits for both parties involved can be described as symbiotic. Chip designers formed a symbiotic relationship with HPC to achieve their goal. The same can be said of developers’ relationship with machine learning systems as the large language models powering generative AI have become more powerful over the past decades.
Like chips, generative AI systems have seen exponential growth. But this growth has been compounded into a briefer timeline, specifically over the past five years. It can be measured either in the computing power required to train the models or in the number of parameters contained by the models, an indicator of their complexity and flexibility. For example, in 2019, OpenAI’s GPT-2 contained just 1.5 billion parameters. In 2022, Google’s PaLM contained 540 billion parameters (Stanford University, 2023). Today, it’s estimated that OpenAI’s GPT-4 contains well over 1 trillion parameters.
Now some of those developing these large models say we should slow down, lest humanity’s symbiotic relationship turn into a predatory one – with us playing the part of prey. This comes after said developers scraped the digital commonwealth of the web in the quest for more data to feed their growing algorithms, in pursuit of continued exponential growth. Many creators – from writers to illustrators to coders – are protesting that their consent wasn’t sought to be a part of these data sets. Several lawsuits before the courts could determine how exactly copyright concepts and training an algorithm intersect.
For IT organizations, the exponential development of generative AI can’t be ignored any longer. Just as Moore’s law pushed demand for constantly higher-performing and always-miniaturizing computing power in the enterprise, IT must now work to enable new AI capabilities. As with the digital age, this will transform enterprises from their back-office operations to their very business models. Simultaneously, IT must prepare a new set of controls that mitigates the risks brought by AI. From securing the new systems to protecting against irresponsible use, IT departments will be asked to supply governance to an area that’s attracting increased attention from regulators and courtrooms.
It’s up to IT to balance the organizational demand to harness AI’s capabilities with the need to protect the organization from the emergent threats posed, to dictate the terms of this symbiotic relationship that’s already in full swing. Welcome to the era of The Generative Enterprise.
IT’S UP TO IT TO BALANCE THE ORGANIZATIONAL DEMAND TO HARNESS AI’S CAPABILITIES WITH THE NEED TO PROTECT THE ORGANIZATION FROM THE EMERGENT THREATS POSED, TO DICTATE THE TERMS OF THIS SYMBIOTIC RELATIONSHIP THAT’S ALREADY IN FULL SWING. WELCOME TO THE ERA OF THE GENERATIVE ENTERPRISE.
Tech Trends 2024: The Generative Enterprise
Seize Opportunities
- AI-Driven Business Models
- Autonomized Back Office
- Spatial Computing
Mitigate Risks
- Responsible AI
- Security by Design
- Digital Sovereignty
Throughout our report, we’ll examine how organizations that have already invested in AI or plan to invest in AI are behaving compared to organizations that either do not plan to invest in AI or don’t plan to invest until after 2024. We’ll refer to these two groups as “AI adopters” and “AI skeptics” for simplicity. Here’s a quick breakdown of what each of these groups look like:
AI adopters: Organizations that have already invested in AI or plan to do so by the end of 2024 (n=430).
- More likely to be from larger organizations, with 37% of respondents estimating a total headcount above 2,500.
- More likely to have a larger IT budget, with 48.5% reporting a budget of at least $10 million.
- 62% located in North America.
- Represent a wide swath of industries including 20% in public sector and 11% in financial services.
- Most likely to rate IT maturity level at “Support” (38%) or “Optimize” (29%).
AI skeptics: Organizations that either don’t plan to invest in AI until after 2024 or don’t plan to invest at all (n=176).
- More likely to be from smaller organizations, with 52% of respondents estimating a total headcount of below 1,000.
- More likely to have a smaller IT budget, with 65% reporting a budget of under $10 million.
- 63% located in North America.
- Represent a wide swath of industries including 26% in government and 11% in manufacturing.
- Most likely to rate IT maturity level at “Support” (42%) or “Optimize” (27%).
We are interested in delineating between AI adopters and skeptics because AI and machine learning (ML) will see the fastest-growing adoption among all emerging technologies in our survey. Nearly one-third of respondents say they plan to invest in AI next year. An additional 35% say they are already invested and plan more investment in AI.
Top five technologies: Organizations not currently invested but plan to invest in 2024
- AI or ML – 32%
- Robotic process automation (RPA) or intelligent process automation (IPA) – 22%
- No-code/low-code platforms – 20%
- Internet of Things (IoT) – 14%
- Data management solutions – 14%
Our emerging technologies quadrant considers existing investment and intended investment for the year ahead as growth indicators, and investment planned for further into the future or no investment at all as stagnation. In this analysis, AI is hot on the heels of transformative technologies like cybersecurity, cloud computing, and data management solutions. The planned investment in AI among those not already invested indicates it has more momentum than any of these other transformative technologies for 2024.
Other noteworthy standouts from the quadrant:
- Mixed reality leads the "not invested but plan to invest after 2024" category at 21%.
- Quantum computing leads the "No plans to invest" category at 81%.
- On-premises servers lead the "Already invested, but do not plan further investment" category, at 33%.
Focus on transformers
We’ll also feature some highlights from another group of "Transformers," or organizations that rank themselves at the top of Info-Tech’s IT maturity scale.
IT Maturity level
Choices | Response % |
IT transforms the business | 14.2% |
IT expands the business | 8.1% |
IT optimizes the business | 28.4% |
IT supports the business | 38.9% |
IT struggles to support the business | 10.4% |
(n=676)
About one in six IT leaders describe themselves as innovators. Most put themselves at either the “Support” or “Optimize” level of maturity.
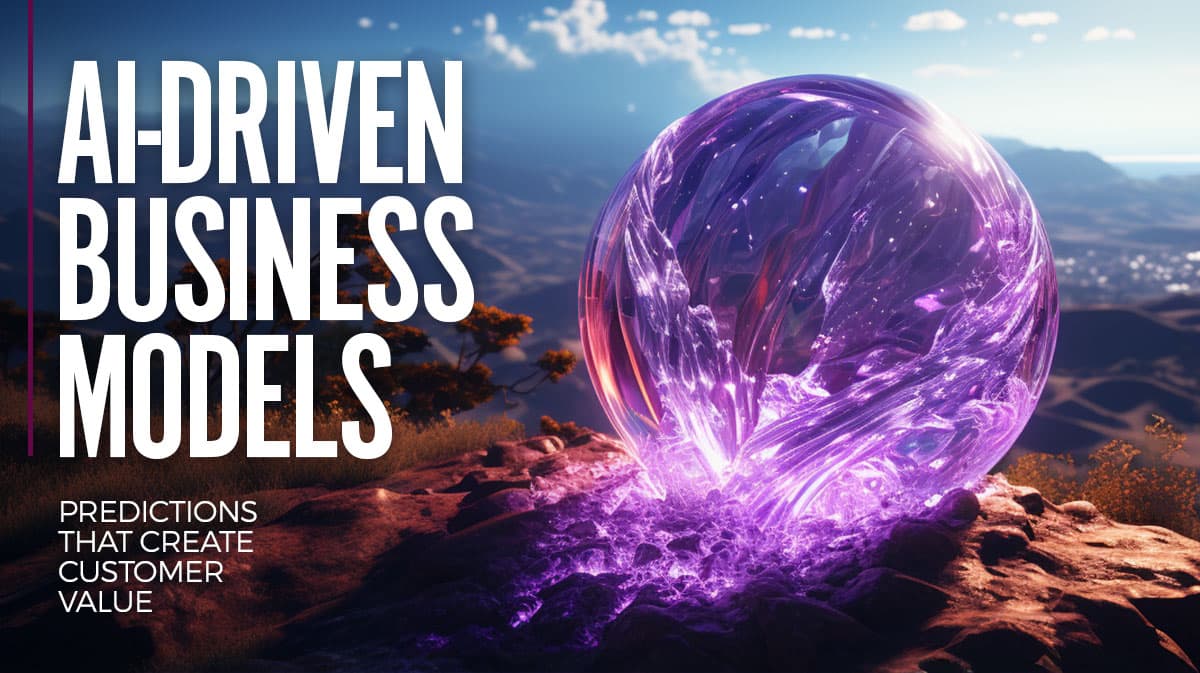
Introduction
Predictions
as products
The commercialization of AI models is based on the value of an accurate prediction. Algorithm builders train their neural networks to make good predictions by using a lot of historical data and sometimes adding in human feedback to help sort out special circumstances. Once trained, the algorithms can make predictions based on new data. It’s a concept that the tech giants of our era have demonstrated for the past decade, with Facebook predicting which ads you’re most likely to find relevant, or Amazon predicting what products you’ll want to purchase next.
More recently, we’ve seen the technology sector move from augmenting its business models (e.g. to sell ads, or as buy-everything e-commerce stores) with AI predictions to making the AI predictions themselves the product. Midjourney is an example of an image generator that predicts what an image should look like based on a user’s prompt. OpenAI’s ChatGPT predicts the right words to respond to a prompt. But selling predictions won’t stop there. As AI becomes more effective, it’s displacing established approaches to solve problems and helping industries solve previously unsolved problems. It’s being used by airports to manage flight control centers, by pharmaceutical firms to research new drugs, and by financial services firms to detect fraud.
According to our survey, most IT organizations are making plans for AI to drive strategic aspects of their business in 2024. It will be uncharted territory for many, and there will be new risks to consider as these new business models are forged.
AI-based business strategies aren’t just for those on the cutting edge. In our Future of IT survey, about one in five IT leaders told us they are already using AI to help define business strategy.
Signals
Investment
is optimism
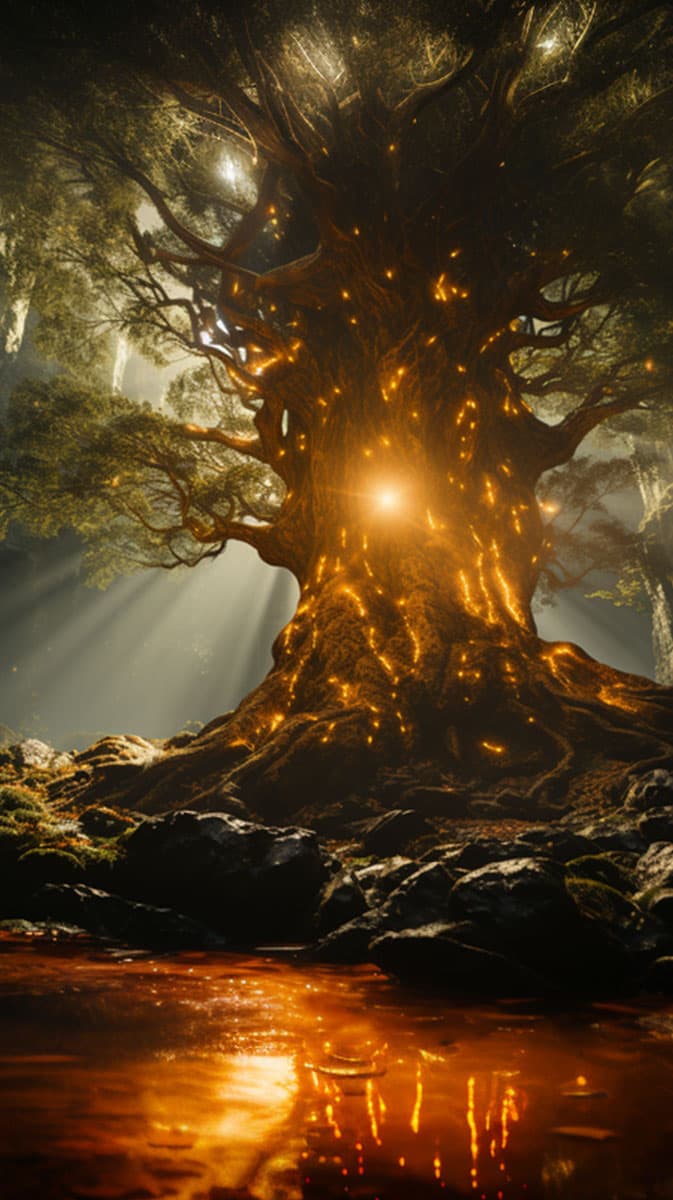
Discourse about AI tends to sway between two extremes – either it will wipe out humanity or it will solve all of our problems; either it will cause mass unemployment or it will free workers from the shackles of tedious minutiae to focus on more valuable tasks. Yet most IT practitioners tend to see AI’s impact as somewhere in the middle, while maintaining optimism overall.
AI adopters are much more optimistic than skeptics. Two-thirds of them say AI will bring benefits to their businesses. But skeptics aren’t doom and gloom either – half are merely on the fence about it, anticipating a balance of benefits and challenges. Only 3% of skeptics feel their business faces an existential threat from AI, and no adopters are in this camp. Transformers are similar to AI adopters in this area, with two-thirds also saying they are feeling positive about AI.
Organizations are making plans for AI to feature in strategy and risk management capabilities. Among AI adopters, “Business analytics or intelligence” is the most popular selection in this category, with more than three-quarters planning to use AI there by the end of 2024. Seven in 10 organizations also plan to use AI to identify risks and improve security by the end of 2024. Since AI skeptics are not investing in AI before the end of next year, most of them skipped this category or indicated delayed or no plans to use AI in any of these areas. But some did indicate plans to use AI in these areas despite a lack of investment. Perhaps they’re hoping to dabble with free trials or have their workers fiddle with open-source models.
Survey
What
overall impact do you expect AI to have on your organization?
Survey
By
the end of next year, what sort of strategic or risk management tasks will
your organization be using AI for?
The Transformers segment stands out here, as they indicate by far the most interest in using AI to define business strategy, with more than two-thirds saying so. Fewer than half of adopters plan to do so.
Opportunities
& Risks
A
high-risk, high-reward scenario
Opportunities
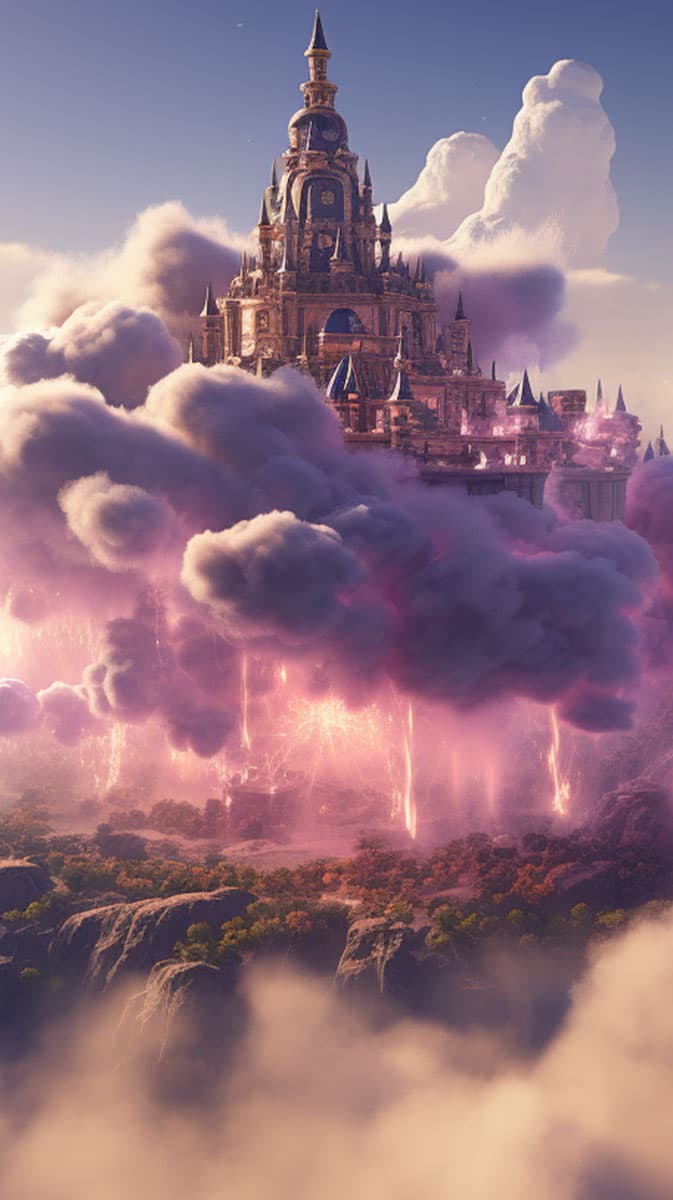
Disruption
Using AI predictions to solve problems that previously required more overhead, or to solve new problems altogether, has the potential to disrupt many different industries. Similar to the digital revolution that saw software take on so many business operations more effectively, AI is quickly becoming an obvious best option for many tasks.
Reduce decision friction
AI can consider many more complex factors in a given situation than a person ever could and boil it down to a simpler choice (Agrawal et al., 2022). This can help organizations provide customers opportunities they might not have taken, or it can empower employees to push ahead with a project.
Scale
Training models is difficult, requiring talented data scientists and access to powerful compute resources. But once a model is fully baked, it can be deployed to edge devices to provide service at very little cost. It’s an upfront capital requirement with low long-term overhead that is easy to scale.
Risks
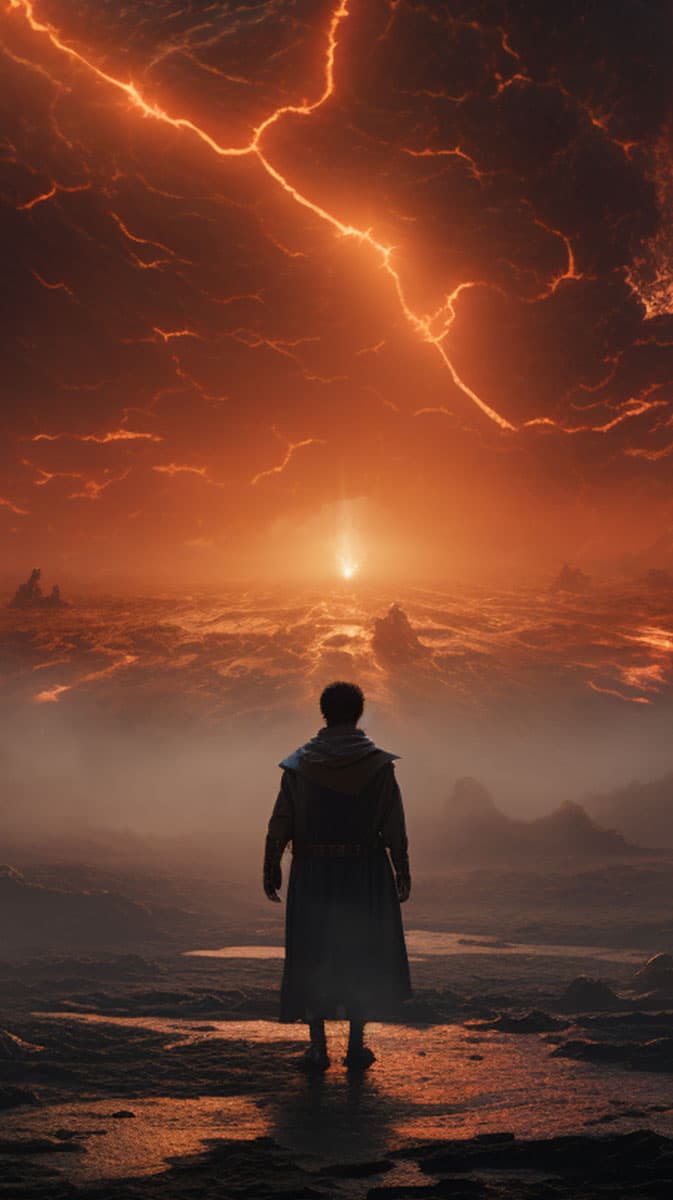
Easy to replicate
After OpenAI made its splash with ChatGPT in November 2022, Meta responded by releasing its model’s code to open source ("Meta Made Its AI Tech Open-Source," New York Times, 2023). This gave developers an alternative path to harnessing the capabilities of a large model without paying to use OpenAI’s APIs. ChatGPT continues to do a brisk business, but already, many more similar chatbots have emerged for use free of charge. With the method of building foundation models commercialized, businesses may find their competitors are able to quickly respond to any competitive advantage with similar updates. Pushing the capabilities to market for free could drive the value of making certain predictions to zero and disrupt a business model.
Rapid obsolescence
Here’s another shortcut a model might take to irrelevance, as technological advancement in this space seems to be a weekly occurrence. Multimodal inputs look to be the next advancement on the horizon, which would make text-only, speech-only, or image-only models seem antiquated only months after creating shockwaves around the world (Meta AI, 2023).
Ethical quandaries
Creators of generative AI models are openly saying there may be a 1 in 10 chance that AI poses an existential threat to humanity. Whether they’re right or not, some will say anyone developing AI capabilities is contributing to the problem. Ethical concerns don’t stop there, as many creators are fighting back against perceived theft of intellectual property. Also, opting to use AI instead of hiring a person to do a job is likely to invite criticism.
We see the visible light spectrum from a light bulb because our eyes are adapted to that frequency of electromagnetic waves. But for Wi-Fi, we’re not adapted. If we could see these signals or interpret them, what would that give us?
Taj Manku,
CEO, Cognitive Systems
Case Study
What does
your router see?
Situation
Working in a previous company that he founded to build chips for cellular phones, Taj Manku often considered how the chips could “see” cellular base stations and Wi-Fi access points in a way that humans couldn’t. Instead of the opaque objects perceived by the human eye, they were illuminating beacons, radiating out electromagnetic signals. What if, the physicist and Ph.D. lecturer at the University of Waterloo wondered, we could give people the ability to see that signal in the same way? In 2014 he founded Cognitive Systems Corp. to find out.
“It spawned from the idea of how can we use this radiation that’s already in your home and how can we build application on this type of technology,” he says in an interview. Cognitive Systems trained an AI system that could sit on a Wi-Fi access point and interpret the signals in a different way beyond the information being transmitted. The AI uses the Wi-Fi fields between the access points and the devices connected to it to understand the environment of the home. Then it can detect when a human moves through that environment, disturbing the signal. A statistical profile is used to detect the unique way a human body partially reflects the signal as it moves.
To sort out human movement from pet movement or a fan, Cognitive Systems used reinforcement learning with human feedback (RLHF), a combination of a computer looking for patterns and a researcher providing feedback about whether it’s correct or not. The model that’s deployed to the edge – in this case, a Wi-Fi access point – can adapt to a changing environment if someone decides to rearrange the furniture.
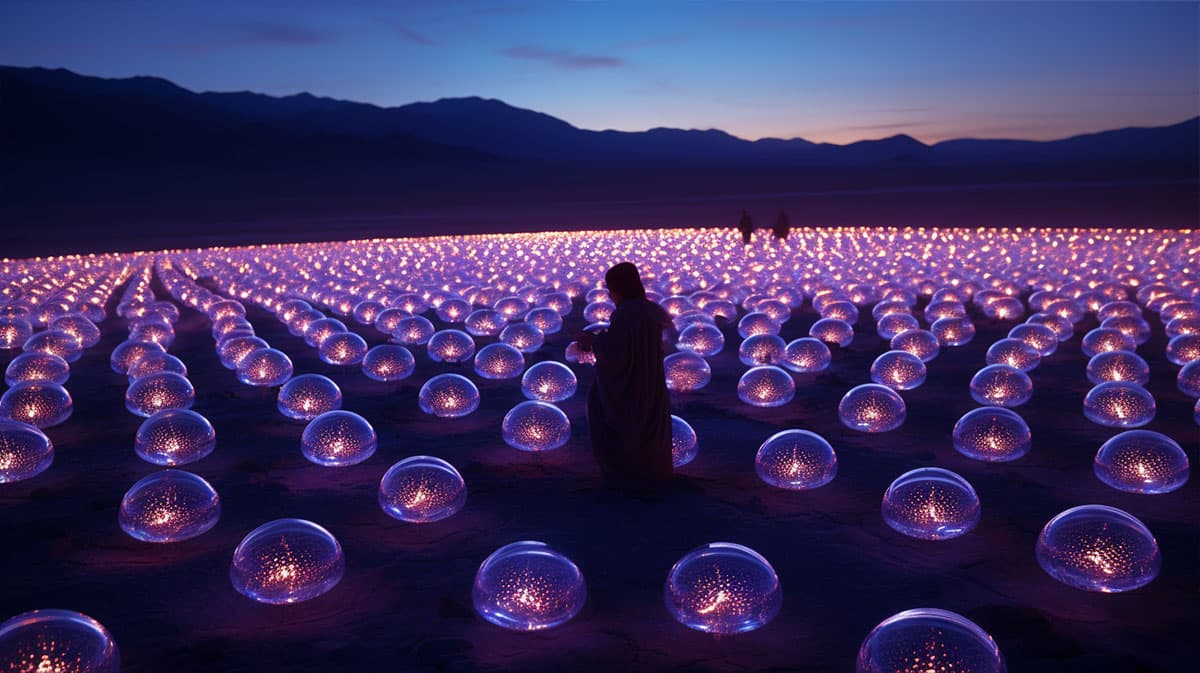
Action
The first go-to-market strategy was to sell the service directly to consumers. But after slow uptake, convincing one customer at a time to fiddle with their router settings, Cognitive Systems pivoted to a business-to-business model as a software vendor. It partnered with chip makers to receive the deep-system access its software – dubbed Wi-Fi Motion – required for installation on routers, and sold the software to internet service providers (ISPs) that could deploy at scale. Cognitive charges ISPs in a SaaS model, creating recurring revenue.
ISPs get a value-added service they can deliver to customers. Typically ISPs only see customers use their applications to pay a bill or resolve a service disruption, so providing a beneficial feature is an opportunity to create a better relationship. The primary value proposition of the service is as a security system that requires no additional hardware. When customers are away from home and don’t expect anyone to be in the house, they can be alerted to the presence of a person.
Once a customer activates the home monitoring service, there are upselling opportunities. A wellness monitoring feature can alert a caregiver when an elderly home occupant hasn’t moved for an extended period, and a smart home automation can adjust the thermostat and turn the lights on or off according to people’s movement through the home and out the door.
Privacy is a priority for Manku, who chooses to comply with the strictest data privacy laws in the world – currently those of the state of California, he says. Customers must opt in to using the software on their access points first. The technology isn’t capable of identifying an individual – it can only detect a person’s movements – and the information isn’t discrete enough to differentiate between someone doing jumping jacks and running on the spot.
Result
Today, Cognitive Systems is deployed to more than 8 million homes and is growing. It sees its revenues double annually. It is working with 150 ISPs around the world and is seeing those ISPs onboard new users of the service every day. Manku offers this advice to entrepreneurs pursuing an AI business model:
“It has to be scalable. I would try to stay away from hardware. I would focus on a software-based solution. Hardware solutions are tougher because you have to deal with a lot of the management of the procurement, and that can be difficult.”
Rather than trying to find one customer at a time, look for an opportunity to find a million customers or more at a time. ChatGPT falls into that category, becoming the fastest growing technology ever by making its service available to anyone via a web browser.
What’s
Next
A model of
imperfection
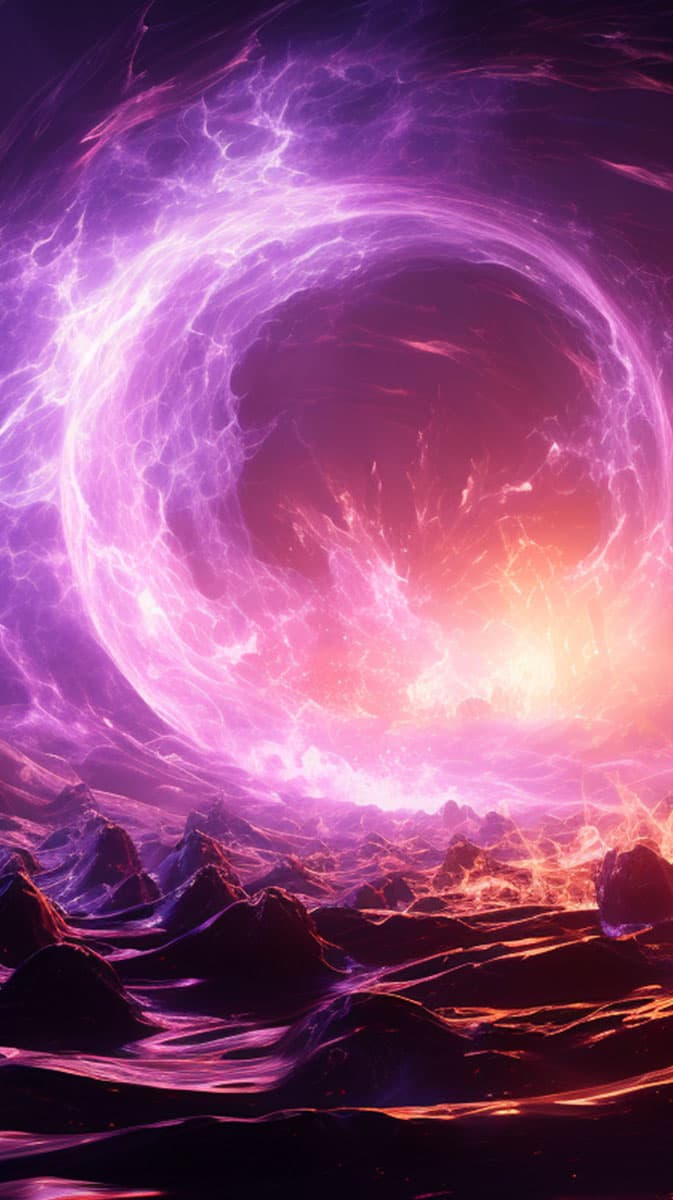
Multi-modal models
If 2023 has been all about LLMs (large language models) then 2024 might be about MMMs (multimodal models). These models will be capable of receiving different modes of input, such as an image, text, or audio, and generating multiple modes of output in turn. Meta’s SeamlessM4T model is an example, combining both text and speech in a model designed to translate between almost 100 different languages. The model supports nearly 100 languages for both speech and text input, can provide text transcription in nearly 100 languages, and can provide speech output in 36 languages (Meta AI, 2023).
Longer-context models
Developers using ChatGPT or its API equivalent often run into a barrier with the amount of specific context that they can provide to the model. It’s a concept that’s referred to as the attention span of the model, and the longer it is, the more useful to enterprises who want to use their own data to guide output. Building a longer attention span is one of the main motivations to train new foundation models, and the cutting edge at the moment is a 32K (or 32,000 tokens, equivalent to about 25,000 words) limit, offered by OpenAI’s GPT-4 model (“What Is the Difference Between the GPT-4 Models?” OpenAI, 2023).
Industry-specific models
While large models are flexible and can be used for a number of different tasks, many industries find these models too unreliable to depend upon. Accuracy isn’t important if you’re using ChatGPT to provide dialogue for a character in a video game, but it is necessary if you’re going to use it to present citations in a courtroom or engineer a new drug. Specific data sets will be required to hone AI enough to make accurate predictions, and even then, humans will need to work to verify results. Early examples of industry-specific models come from the legal industry, where Harvey AI, CoCounsel, and LitiGate all compete to offer law firms AI services. Similarly, in the pharmaceutical industry, not only is AI helping design new drugs in the lab and predict their likelihood of approval by regulators, but it is also helping monitor clinical trials by interpreting sensor data (“AI Poised to Revolutionize Drug Development,” Forbes, 2023).
Recommendations
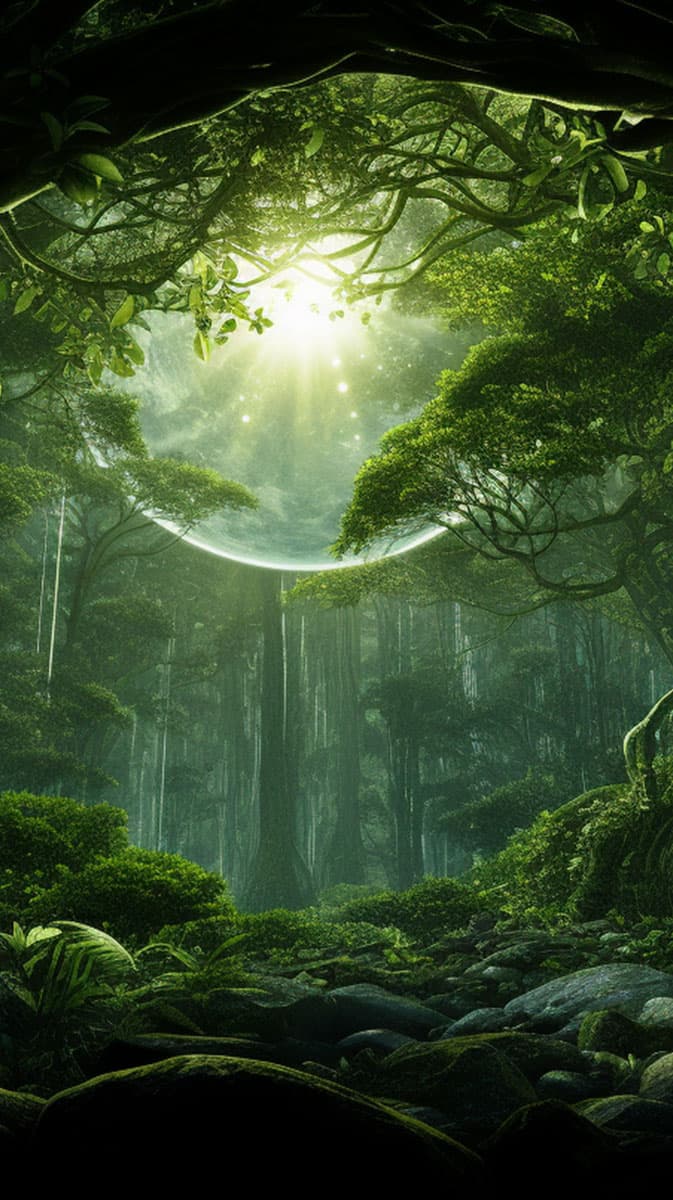
Align with your business stakeholders on opportunities to provide customer-facing value with AI. Primary considerations will be what problems your customers are trying to solve, where they face friction with your products and services at present, and what data you own that can be harnessed to train a model. Building a pilot project to test out new ideas in the real world is desirable, rather than try to transform the entire business all at once.
Info-Tech Resources:
Build Your Generative AI Roadmap
Generative AI has made a grand entrance, presenting opportunities and causing disruption across organizations and industries. Moving beyond the hype, it’s imperative to build and implement a strategic plan to adopt generative AI and outpace competitors.
Yet generative AI has to be done right because the opportunity comes with risks, and the investments have to be tied to outcomes.
AI Trends 2023
As AI technologies are constantly evolving, organizations are looking for AI trends and research developments to understand the future applications of AI in their industries.
Build Your Enterprise Innovation Program
Collect ideas from business stakeholders in a constructive way and prioritize initiatives that could be worthy of a pilot project.
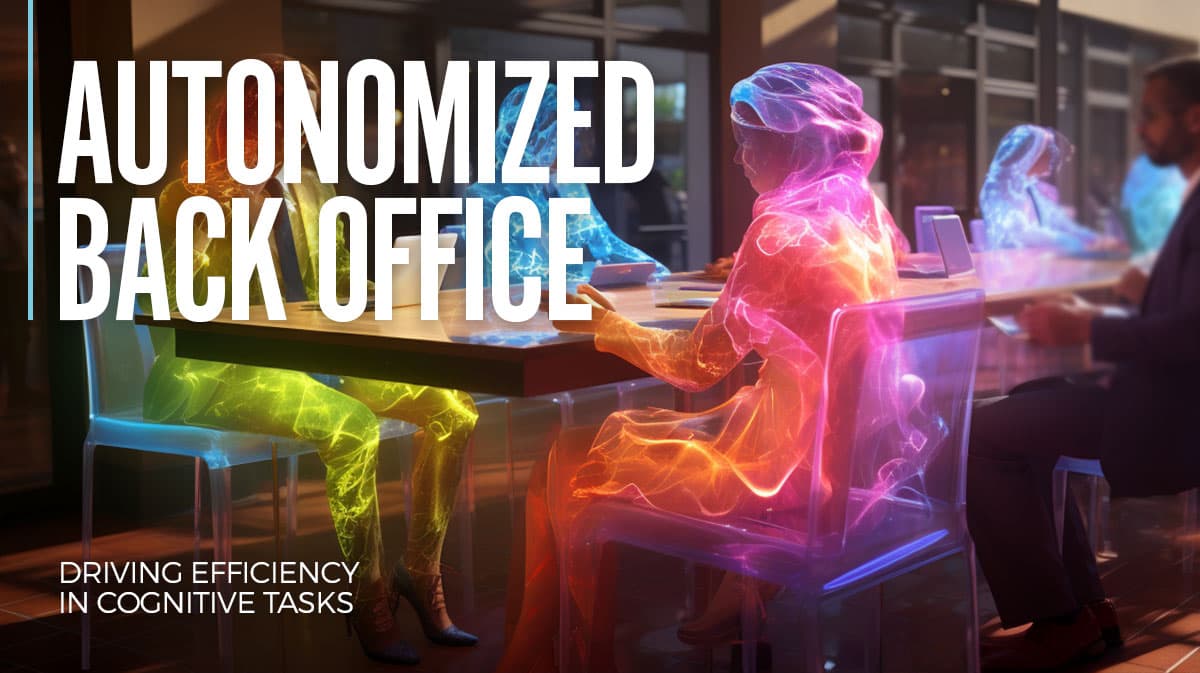
Introduction
Enterprise software gets chatty
IT’s role has always been to autonomize business systems by providing capabilities that allow systems to self-execute and self-regulate toward company goals in the name of efficiency. With generative AI, a wide range of new tasks become possible to automate toward this goal. These AI models are adaptable and flexible, able to process large volumes of unstructured data and provide classification, editing, summarization, new content creation, and more. Consultancy McKinsey estimates that, by automating these routine cognitive tasks, generative AI’s impact on the economy could add $2.6 to $4.4 trillion in value across 63 use cases – and double it if generative AI is embedded into software already used for other tasks beyond those use cases (McKinsey, 2023).
So even for organizations not transforming their business model around AI, there will be value to reap from streamlining current operations. Some of this increase in efficiency will be delivered by using new applications or web services, such as ChatGPT, but much of it will be delivered through new features in software that’s upgraded with new AI-powered features. With the software as a service (SaaS) model, in many cases, enterprises won’t even need to deploy an upgrade to harness these new features. Existing vendor contracts will be the most likely avenue to add generative AI to many enterprises’ IT arsenal. The list of vendors that have announced generative AI features is too long to include here, but consider several examples of vendors in the IT space alone:
- Juniper Networks announced integration of ChatGPT with Marvis, its virtual network assistant. The chatbot will be better at helping users review documentation and provide customer support to resolve networking issues (Juniper Networks, 2023).
- CrowdStrike released Charlotte AI to customer preview, its own generative AI that answers questions about cybersecurity threats and allows users to use prompts to direct the automation of repetitive tasks on the Falcon platform (SDX Central, 2023).
- ServiceNow announced the Now Assist assistant for its Now platform, which automates IT service workflows. The assistant summarizes case incidents. Another feature allows developers to generate code with text prompts (CIO, 2023).
In other lines of business, major vendors like Microsoft, Salesforce, Adobe, and Moveworks are among those announcing generative AI features. Generative AI is going to impact all industries, but some sooner than others. As we’ll see in the case study, the legal industry is one where generative AI solutions are more specialized and deployed among early adopters.
First, we’ll examine how organizations plan to approach new generative AI features from vendors.
Signals
It’s either roll out or opt out
Many generative AI features will enter the enterprise through feature upgrades to existing business applications. In some cases, IT may have the keys to the admin controls, and in other cases, it will rest with the line of business that procured the solution. For SaaS solutions that bolt on generative AI chatbots and other features, IT may find that they are turned on by default with new versions, and action is required to opt out of them.
If given the choice, nearly half of adopters (47%) are keen to adopt new generative AI features from major vendors either in beta access (17%) or when generally available (30%). The other half are still taking a more cautious approach, with 37% saying they need more information before deciding and 16% saying they will hold off on the features until other organizations test them.
Skeptics are about twice as likely as adopters to say they need more information or are not interested in adopting generative AI features at all. Less than 1 in 5 skeptics say they will be adopting new generative AI features at general availability or sooner.
Survey
With
business application providers planning to upgrade their software with
generative AI features (e.g. Microsoft Copilot, Adobe Firefly), how do you
plan to manage the rollout of these features?
What back-office jobs will AI do?
We asked what type of operational tasks organizations are most interested in using AI for. One in three adopters say they are already using AI to automate repetitive, low-level tasks. Another 45% say they plan to do so in 2024. More than a quarter of adopters are also already using AI for content creation (27%), with another 30% saying they will do so in 2024. More than one quarter of adopters say they already use AI for IT operations (27%), and 42% say they will use it for IT operations in 2024. Applying AI to IoT and sensor data generated the least interest among adopters, with 41% saying they had no plans to use it.
Skeptics aren’t likely to have adopted AI for any operational tasks yet, but they are more likely to leave room for adoption rather than close the door on it completely.
There’s one thing that most adopters and skeptics seem to agree on – they are more interested in seeing AI automate tasks rather than augmenting operational staff in their decision making. Almost 1 in 5 adopters say they have no plans to pursue augmenting staff with AI, and 46% of skeptics say the same. This seems to run contrary to the message that many businesses and vendors often say about AI: that it is intended not as a replacement for people doing jobs, but as an augmentation.
SURVEY
By the end of next year, what sort
of operational tasks will your organization be using AI for?
OPPORTUNITIES & RISKS
Seize Opportunities and Mitigate
Risks
Opportunities
Cost savings and efficiency gains
With more cognitive tasks automated, employee time can be spent on higher-value tasks, or less overhead may be required to manage a process. Organizations will be able to scale to support more business without being bogged down by administrative nickel-and-diming, though using generative AI will represent a new cost in itself.
Improved output
By getting to a first draft more quickly, workers can spend more time honing their message and putting a point on the finer details. Using generative AI to augment workers is often a path to improved quality and modest time savings.
Ease of access
With major enterprise vendors eager to compete in launching new generative AI features, the new capabilities may be rolled in as a value-added component to existing contracts. Organizations can work with vendors where they’ve established a trusted relationship.
Risks
Hallucination situation
Even when trained on specific data sets and built for purpose, generative AI is still prone to fabricate information and present it as fact. Knowledge workers using outputs from generative AI tools will need expertise to validate facts provided by these tools (Interview with Monica Goyal).
Data security and privacy
Old fears about third-party hosts getting access to sensitive data will be revived. Organizations using generative AI features on hosted software will perceive new risks around their data being used to train the vendor’s algorithm. Vendors will commit to not doing so in contracts, but risk managers will point out it’s still technically possible. New features may be blocked in some situations by cautious administrators.
Ethical liability
Employees who don’t grasp the limits of AI’s capabilities may be over-reliant on its output or try to use it for a task that’s not appropriate. Governance of new AI capabilities will require training for users to avoid inadvertent or intentional cases of ethical misuse of AI.
AI “is going to give us more certainty in how long things take to do, and that's going to allow us to do more fixed-price billing.”Monica Goyal,
Lawyer and Director of Legal Innovation, Caravel Law.
Case Study
ChatGPT passed the bar exam; now it’s working in law
Situation
When researchers found that OpenAI’s GPT-4 could not only pass the bar exam, but do so in the 90th percentile, it made it seem like AI lawyers were just around the corner (ABA Journal, 2023). But that notion took a hit when a New York City lawyer submitted a legal brief created by ChatGPT that was full of fake legal citations, with the lawyer claiming he did not comprehend that ChatGPT could fabricate cases (“The ChatGPT Lawyer Explains Himself,” The New York Times, 2023).
Despite that widely covered inauspicious introduction to the courtroom, AI still has the potential to transform the legal industry. AI can augment a lawyer’s expert-level capabilities by providing first drafts of legal content, translating technical legal language into more colloquial terms for clients, and reviewing contracts or agreements. In one analysis that included data from 10 corporate legal departments, researchers found that 40% of time entries representing 47% of billing could potentially use generative AI. Given an upper limit of generative AI to reduce that work by half, law firm revenue could be reduced by 23.5% (3 Geeks and a Law Blog, 2023).
Technology companies have already been launched to provide generative AI tools that are specifically trained for the legal industry. These tools are trained on case law that sits behind the paywall. Competing vendors in the space include Harvey.ai, Casetext’s CoCounsel, and Litigate.ai. Other applications, like Rally’s Spellbook, also apply OpenAI’s GPT-4 to more pointed tasks, such as contract review and drafting (Interview with Monica Goyal).
As with many commercial applications, it’s still early days for AI in law. But at Caravel Law, Monica Goyal, lawyer and director of legal innovation, is implementing the technology to see where it can optimize the operations of this Toronto-based non-litigating practice.
Action
Caravel is an early user of Harvey, which is not yet generally available. Based on OpenAI’s GPT-4, Harvey also received funding from OpenAI to pursue a solution for the legal industry (Global Legal Post, 2023). Harvey works similarly to ChatGPT, offering a simple chatbot interface that allows users to enter prompts.
“If you have some training around what is a good user prompt, you will get a better result,” Goyal says. “The more fulsome you can be, the better the output.”
Lawyers can ask Harvey legal questions about particular areas of law. Caravel’s lawyers have learned to specify that they are interested in Ontario-based law, and they find that improves the results. Harvey also has a document upload feature, so a user can submit a PDF or an email as context and then have Harvey draft a document such as a notice or client correspondence. The output will not only lean on its trained model, but also reference the uploaded documents with citations.
Caravel is also using Spellbook by Rally, which is a Microsoft Word plug-in that uses GPT-4 to review contracts and suggest additions. It’s also looking to improve other back-office operations such as business development support. Goyal’s team customized Julius.ai to allow their business development representatives to query it about lawyers’ skillsets and availability for new clients.
Goyal leads a tech innovation team that is assembled based on the project requirements. To customize Julius, she contracted short-term employees. In other situations, she’s partnered with a vendor or hired a consultant.
Caravel finds the technology effective overall but cautions that it’s still prone to make mistakes, like citing case law that doesn’t exist, and that its output must be validated. Caravel’s lawyers tend to have 10 years of experience or more, and Goyal worries that a less experienced lawyer wouldn’t have the expertise to properly validate output from generative AI tools. “If you have less than five years of experience, you might not do very well to verify it’s accurate,” she says.
Result
After accounting for the additional validation of output, Goyal estimates Caravel lawyers are saving about 25-50% of their time spent on creating a legal draft. In the case of Spellbook, lawyers are saving more like 10-15% of their time on contract reviews.
“It’s going to save you some time, but you have to go through the contract and make sure that you read it and that you know it,” Goyal says. But using generative AI isn’t just saving the lawyers time – it’s also about creating a better output in the end.
Harvey commits to its customers that it will not use their data to train their model. Goyal says this is sufficient assurance for protecting sensitive data.
Goyal cautions that in-house legal practices should have clear processes in place before trying to deploy new AI solutions. It’s also important to understand how the software works and what its limits are, recruiting help through vendors or consultants if necessary. But she’s confident the value can be realized by those who understand it.
As a result, law firms are talking about reevaluating the billable-hour model. “People have been talking for a long time in the industry about how the billable model doesn’t work for clients,” she says. “They really don’t like it.” Lawyers had to use the model because their services are so bespoke, and it’s uncertain how much time will be required for services. But with generative AI providing more streamlining, lawyers could be more confident about cost certainty and do fixed-price billing in certain scenarios.
What’s Next
Differentiation comes from the foundation
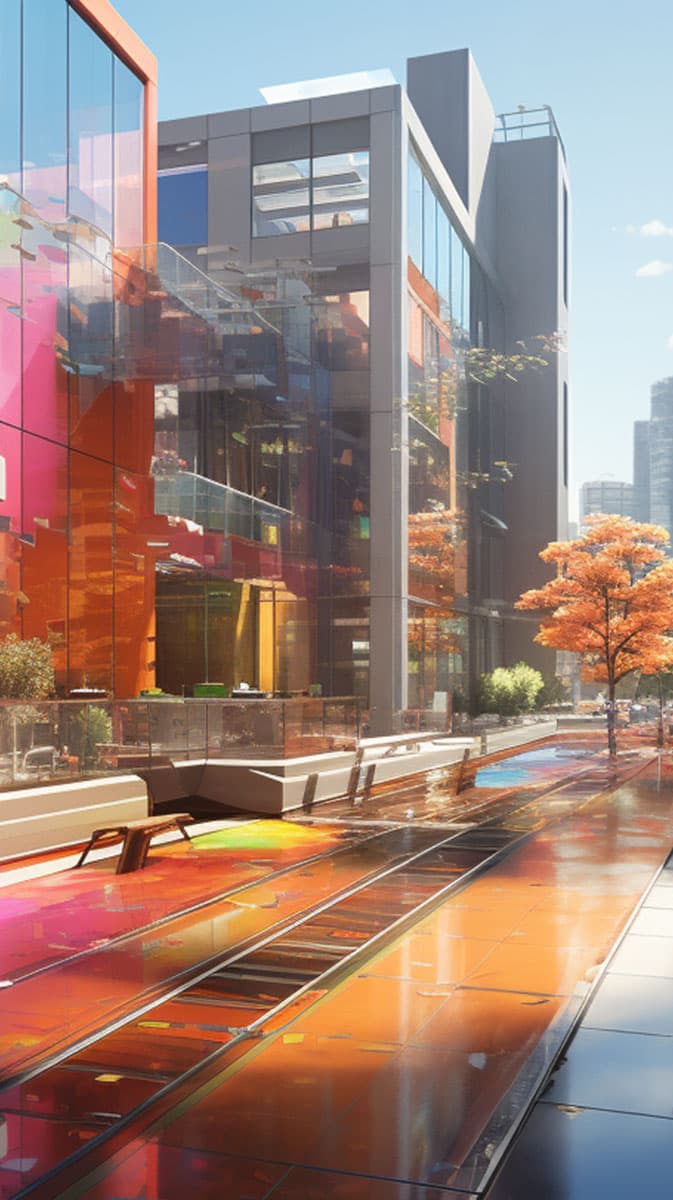
Vendors releasing generative AI features will either be partnering with an AI-focused company such as OpenAI to provide a foundation model, or they will train their own models. Savvy technology purchasers will set aside vendors’ promises about the benefits of these software features and consider the pros and cons of both approaches:
- Vendors that integrate a third-party AI model will have to answer questions about whether customer data is exposed to that party. But the foundational model itself may be more flexible and provide more utility due to being created by an AI-focused firm. There is the risk that the model provider could go out of business or run into regulatory trouble with their algorithms, and that this could affect the performance of the vendor’s solution.
- Vendors that train a proprietary model will have to answer questions about whether they themselves are using customer data to train their own AI models. Customers will want the option to consent to such an arrangement and will expect sufficient value in return. Models are likely to be more purpose-built and less flexible.
There is yet a third, hybrid approach to consider in which a vendor starts with a foundation model provided by an AI company but customizes the model and licenses the rights to host it on their own infrastructure. In our case study, Harvey.ai is an example of this hybrid approach, adapting OpenAI’s GPT-4 model with financial backing from the company as well.
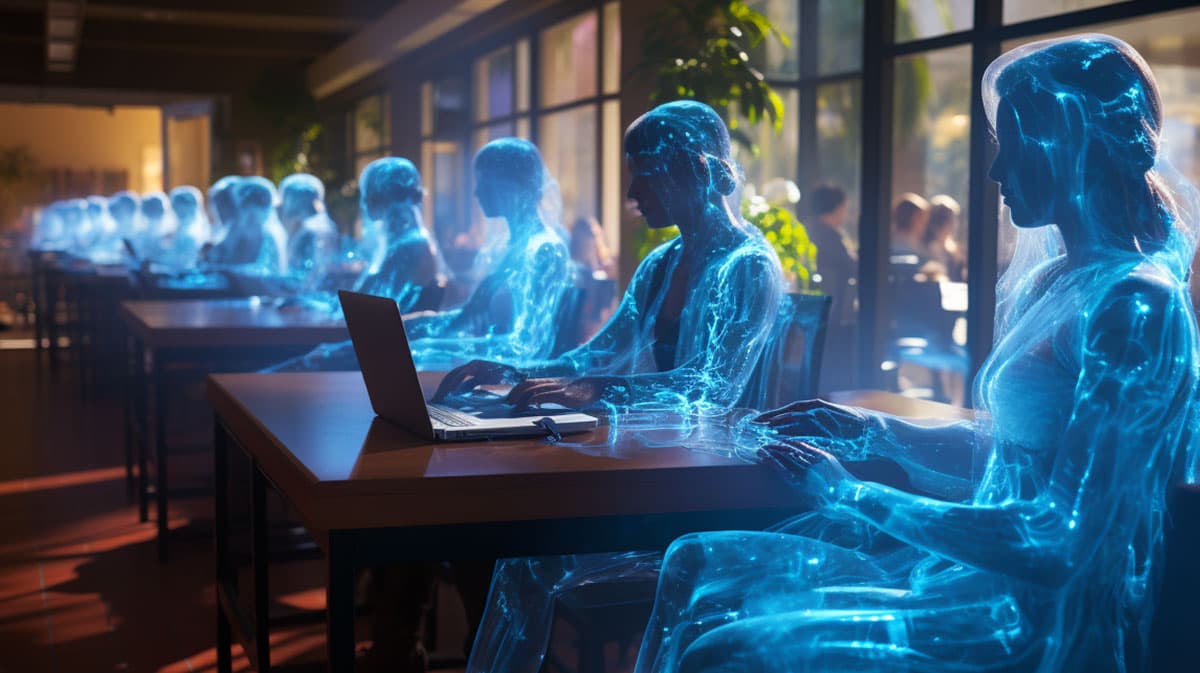
Shadow AI
Following the release of ChatGPT’s beta to the web in November 2022, many organizations quickly deployed policies telling employees not to use the tool. One survey by BlackBerry found 75% of organizations were considering or implementing a ban (BlackBerry, 2023). Yet actually preventing its use is difficult to enforce since it’s free to use and only requires a web browser.
We might expect a similar situation when vendors begin rolling out their own chatbots and other generative AI-powered features. IT departments will need strong governance models to enforce limitations on accessing new features they aren’t comfortable with. At the same time, overly strict limitations on using these new features will give business departments an incentive to cut IT out of the equation and go directly to vendors. Establishing the risk tolerance and specific no-go areas with top level leadership is going to be an important step in effective governance.
Recommendations
Organizations should look to their trusted vendor relationships for opportunities to harness new generative AI features in the tools they are already familiar with. CIOs should keep apprised of new feature releases and any changes to terms of use that come along with them. Once they are satisfied there is no additional risk introduced around sensitive data, there are two paths to pursue for value realization. A pilot project that identifies a specific use case for new features can be selected and launched, or business users can be educated about new features and left to incorporate them to improve their own productivity.
LOOK FIRST TO YOUR TRUSTED VENDORS FOR NEW GENERATIVE AI FEATURES IN TOOLS YOU ARE ALREADY FAMILIAR WITH.
Info-Tech resources
Improve IT Operations With AI and ML
Prioritize IT use cases for automation and make a plan to deploy AI capabilities to improve your IT operations. Calculate return on investment for solutions and create a roadmap to communicate a deployment plan.
Govern Office 365
Prepare for the new generative AI features coming to Office 365 by aligning your business goals to the administration features available in the console. Apply governance that reflects IT’s requirements and control Office 365 through tools, policies, and plans.
Establish a Communication and Collaboration System Strategy
Cut through the redundant and overlapping collaboration applications and give users a say in how they want to work together and what tools they can use. The impact is reducing shadow IT and the burden on application maintenance.
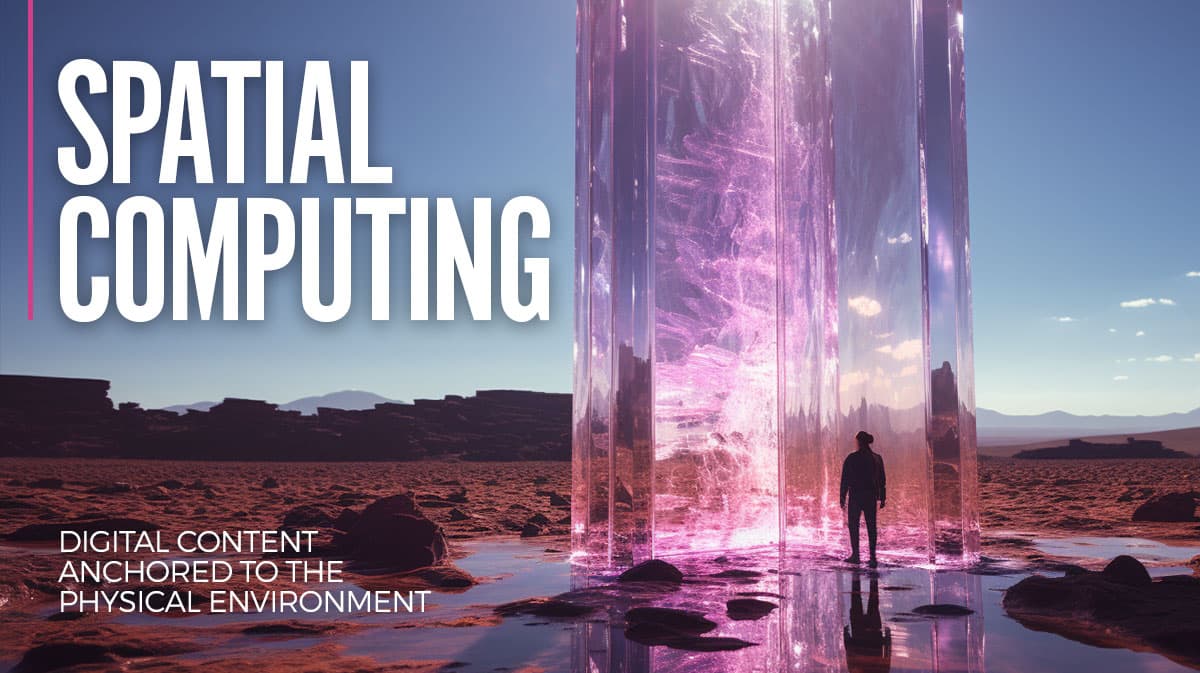
Introduction
From the metaverse to spatial computing
When Apple debuted its Vision Pro mixed reality headset at its Worldwide Developers Conference (WWDC) in June 2023, it had to explain how headset users could participate in videoconferencing. Joining a Zoom call with a phone or a laptop provides a natural place for a camera to point at the user’s face, but once they start wearing a headset, that’s lost. To solve this problem, Apple demonstrated that the Vision Pro’s front-facing cameras and sensors could be used to scan the user’s shoulders and head, then AI would generate an accurate likeness in the form of a digital avatar complete with natural facial expressions (TechCrunch, 2023).
The demonstration shows how AI will be an important part of mixed reality’s mass commercialization. It’s something that Meta also understood, previously sharing plans for virtual assistants that could help headset users cook with augmented reality by identifying where ingredients were in the kitchen or alerting them that they haven’t added the salt yet. Also, an assistant capable of receiving voice commands and rendering fully immersive scenes would be part of a virtual reality experience. While Meta called its vision for this future of computing “the metaverse” and Apple chooses “spatial computing” instead, they are both using the same technological building blocks and converging them to an experience that adds up to more than the sum of its parts.
Both visions are also nascent in development, with Apple expecting to sell well under half a million of its first-generation Vision Pro (“Apple Reportedly Expects To Sell,” Forbes, 2023). In the meantime, generative AI will begin to feature as an interface more often in traditional computing experiences. Voice assistants like Siri and Alexa are being improved with large language models, and just about every major enterprise application seems to be announcing plans for a chat bot addition. Mobile apps capable of scanning rooms and objects and converting them into 3D models are already available on app stores.
At the same WWDC where it announced the Vision Pro, Apple also announced capabilities for iPhones to take photos of a completed meal and provide the user with the recipe. Even Apple understands that mass adoption of mixed reality headsets may be over the horizon, but AI-powered interface advancements can still power spatial computing experiences through already ubiquitous devices.
Most will wait and see if mixed reality lives up to the hype. At least in the meanwhile, many will be exploring generative AI interfaces that will open the door to more spatial computing applications even without the aid of a headset.